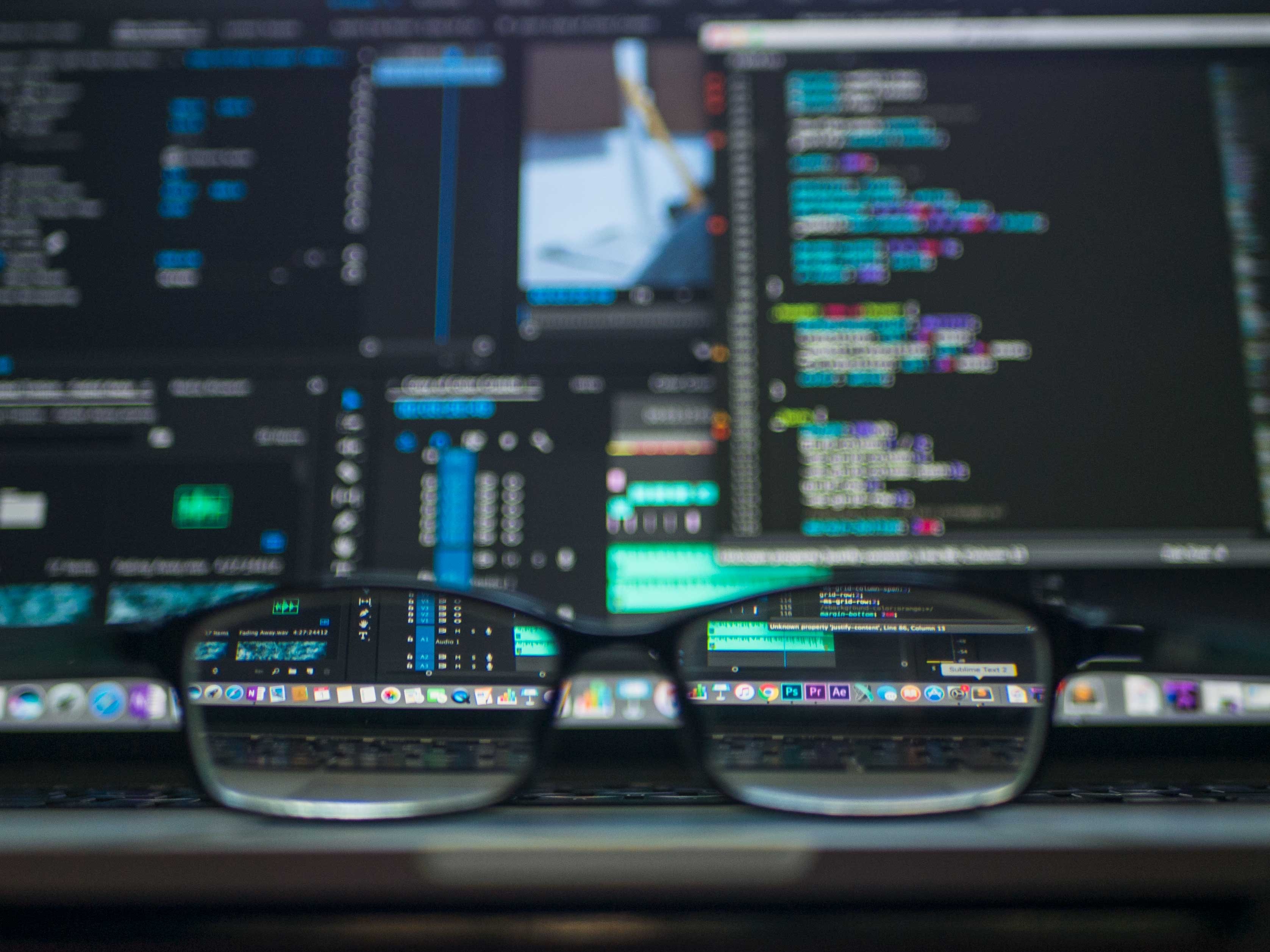
OpenAI's ChatGPT has taken the world by storm since its launch in November 2022. It has become a mainstream tool used by millions on a daily basis that consult it for a wide range of questions.
Powerful as it may be, it is important to ask the question - how far is ChatGPT actually from human experts? And how worried should we be about potential negative impacts of ChatGPT, in particular when it is used in sensitive domains, such as medical or legal?
Today, we will look at a recent paper that focuses exactly on this topic. The paper studies the characteristics of ChatGPT’s responses, comparing its outputs to outputs produced by humans.
So, let's get started!
We also published a video on this topic, you can check it out here.
Introduction
ChatGPT is a dialogue model fine-tuned from the GPT-3.5 series using Reinforcement Learning from Human Feedback, which allows it to perform fluently on a variety of challenging NLP tasks such as story generation and open domain question answering. The surprisingly strong capabilities of ChatGPT have raised many interests, as well as concerns.
On the one hand, people are curious about how close it is to human experts. Does ChatGPT have the potential to become a daily assistant for general or professional consulting purposes?
On the other hand, people are worried about the potential risks brought by large language models like ChatGPT. The free demo of ChatGPT has gone viral, causing a flood of generated content on platforms, threatening their quality and reliability. Some platforms, such as StackOverflow, have banned ChatGPT-generated content due to concerns of low accuracy. There is also a risk of generating potentially harmful or fake information. Performing a detailed and robust evaluation of ChatGPT is therefore very important, for the NLP community, as well as for society as a whole.
Evaluation Dataset
The paper we are looking at today aims to investigate some of these concerns. It does this by collecting a dataset of 40,000 questions and answers produced by human experts and by ChatGPT. The questions are collected from publicly available question answering datasets and Wikipedia text sources on different topics, such as medical and finance. The human answers are obtained either from experts on the specific topic or from high-voted answers on the social media websites from which they come from, such as Reddit.
Turing-like tests
The authors conduct a few Turing-like tests using the dataset. They compare the capacity of human judges to distinguish the ChatGPT answers from the human answers. Two categories of judges are used: 1. experts in the specific field, and 2. amateurs, who have some knowledge, but are not experts.
So, here are the main findings from this study.
When comparing the answers produced by ChatGPT to the human answers side-by-side, it was easy for the judges to distinguish which answer was machine-generated in over 90% of cases. This leads to suggest that ChatGPT outputs have a pattern that is clearly distinct from human outputs.
When the judges are provided with only a single answer, and have to distinguish whether the answer was machine or human generated, the judges were easier to fool. In particular, the amateur experts were fooled over 60% of the time for some of the easier topics.
Another interesting finding is the helpfulness of the responses. The judges were asked to pick which response was more helpful: ChatGPT or human. Surprisingly, the judges rated ChatGPT's responses to be more helpful than human responses in over 50% of cases. That is, apart from the questions in the medical domain. ChatGPT's answers were more concrete, comprehensive, and specific than human answers. For medical questions, however, the judges found ChatGPT's answers to be too lengthy.
Linguistic analysis
Another interesting part of the paper is the linguistic analysis that was performed on the aswers produced by ChatGPT and humans. The authors of the paper asked volunteers to describe the characteristics of both, and then summarised the common themes and differences among all participants.
Here are the key findings they report:
1. ChatGPT's outputs were found to be more objective, focus on the specific question, and usually offer a long and detailed answer that is formal in style. Humans on the other hand were more informal, and tended to easily deviate towards unrelated topics. Humans also used more subjective expressions and expressions that convey more emotions than ChatGPT.
2. ChatGPT sometimes refuses to answer questions it doesn't know. It also has a very strong filter against bias and harmful information. This is good, however, it doesn't always work, since ChatGPT still sometimes fabricates facts when providing a response. This can be a major problem for many use cases, and is definitely something that needs to be investigated and quantified.
All of these findings lead to suggest that ChatGPT might indeed already be useful for many applications and domains, where it can produce helpful and specific responses. There are also several areas where there is still room for improvement, in particular for areas that require focused and specific responses, or factual correctness.
Conclusion
So to sum it up. The paper provides some interesting insights into how ChatGPT compares with human experts, confirming concerns of factual correctness raised by the public. ChatGPT is already very useful for a large percent of questions, domains, and use cases. What remains to be optimised is the tail of the distribution of inputs and responses, which is actually the most difficult part to get right.
There are a few limitations of the paper to mention. For one thing, the datasets they collect contain mostly open questions and answers from forums, rather than answers produced by human domain experts. That is - except for medical, where ChatGPT actually is found to be least helpful by experts. It would therefore be insightful to perform similar evaluations on datasets that are more specialised. Furthermore, the authors didn't perform extensive prompt tuning when calling ChatGPT, which could certaily be useful for alleviating some of its problems. Still, the paper provides interesting insights that help us move closer towards better understanding the limitations and strengths of large language models.
Thanks for reading! If you are looking for state-of-the-art expertise in Natural Language Processing, you should check out our services at The Global NLP Lab.